MCP: Special issue on multiomics
What can you learn from two omes that you can’t tell from one?
You might determine how different bacterial strains in a water sample contribute specific functions to its overall microbiome. You might find that duplication of a section of a chromosome in cancer cells has wide-reaching effects on important proteins — or that it has a smaller effect than expected.
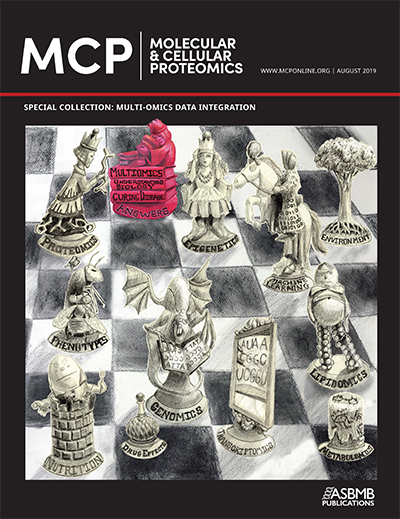
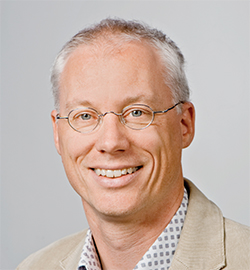
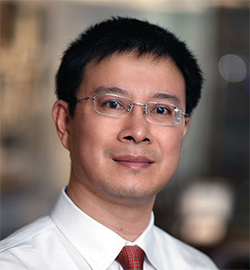
First, though, you need to find a way to wrangle gigabytes of data saved in numerous, perhaps incompatible formats. As high-throughput analytical tools improve, allowing researchers to collect ever more data, the challenge is how to interpret it all.
When transcriptomic, genomic, metabolomic and proteomic analyses are layered together, parsing out a signal can be a monumental task. Data are collected in different forms: RNA counts, genotypes, and mass spectra that might represent proteins, posttranslational modifications, complex carbohydrates or metabolites. To condense this information into coherent, interpretable results the field needs new analytical strategies and new user-friendly software.
Researchers surfing this multiomics wave report a plethora of new tools and approaches in a special issue of the journal Molecular & Cellular Proteomics. The issue, edited by of the Technical University of Munich and of the Baylor College of Medicine, includes 16 articles that explore ways to combine data from two or more omes.
First things first
For readers unfamiliar with multiomics, and colleagues at New York University covers different ways that proteomics data and other types of data can be layered and the biological questions one might answer using these approaches.
An article by of Pacific Northwest National Laboratory and colleagues discusses the importance of data science solutions (such as publishing software and statistical approaches) for reproducibility in handling large datasets from multiomic studies.
Also in the issue:Cancer proteogenomics
A robust section of the special issue includes combined genomic and proteomic approaches to understanding cancer. A number of these studies use a data set from the National Cancer Institute’s Clinical Proteomic Tumor Analysis Consortium and The Cancer Genome Atlas, which make deep proteomic and genomic data from patients with defined cancer types available for bioinformatics analysis.
across the United States and South Korea investigated how copy-number variations affect cellular phenotypes through protein and phosphoprotein abundance. They discovered new genome regions that affect the abundance of important cancer-associated proteins.
and colleagues at Mount Sinai, the University of Chicago and the University of Colorado asked similar questions, integrating transcriptome, phosphoproteome and proteome information about advanced ovarian cancer to understand how copy-number variation or methylation at a given locus can reverberate through the cell.
of Shenzhen University and colleagues in China and the United States combined multiomics with clinical outcomes and images of breast cancer biopsies to yield markers, such as cell density or size, that might be prognostic — a boon for patients, since images of biopsies can be taken at many clinics, while omics approaches are less widely available.
of New York University and colleagues used independent component analysis, a machine-learning approach, to find patterns in breast cancer proteogenomic data sets that might point to new cellular mechanisms.
and colleagues at MD Anderson Cancer Center introduce a new iteration of The Cancer Proteome Atlas, a repository of protein array data from some 8,000 patients’ tumor samples. The new platform, version 3.0, allows users to integrate the available protein array data with other omics data.
and colleagues at Pacific Northwest National Laboratory combined protein and phosphopeptide abundance across tumor samples to identify new kinase substrates and match modifications altering kinase activity to the substrates they affect.
Ome-agnostic approaches
Gene set enrichment analysis, a staple of omics research, involves looking for patterns in the molecules that are altered across conditions.
Two research groups, of the Technical University of Munich and colleagues and and colleagues at Baylor report strategies for combining gene set enrichment analyses across different omic measurements of the same samples.
Metamultiomics: understanding the proteome of the microbiome
Combining multiple omes is challenging enough when working with a defined whole genome that determines the possible array of proteins. But that’s not available for most organisms and even less so for complex microbial communities.
and Indiana University colleagues introduce an analysis program for metaproteomics that measures all of the proteins in a microbiome. The program uses metagenomics and metatranscriptomic data to assemble a custom metagenome specific to the experimental context — for example, ocean or wastewater samples — and then uses that to identify proteins.
of the University of Minnesota and colleagues present software that allows quantitative comparison between conditions in metaproteomic studies and also lets researchers ask how different bacterial groups contribute to functions of the microbial community.
Interactomics
Once the proteins in a sample are described, understanding how they interact gives a deeper insight into their function — and can generate some surprises.
and other researchers at the European Molecular Biology Laboratory investigated how, in cancer with duplicated or deleted sections of genome, protein–protein interactions buffer the final quantity of these proteins encoded in these regions.
and colleagues at Princeton used proteomic and transcriptomic data sets to identify important proteins in the interactome of a Huntington’s disease–associated protein.
Computational tools
Two articles are more specific to proteomics.and other researchers at Ghent University in Belgium introduce a new release of their popular PROTEOFORMER software, which uses ribosome profiling to identify new proteoforms and predict their fragmentation patterns.
and colleagues at the University of Madison report on a web-based tool to visualize and annotate peptide tandem mass spectra no matter what techniques were used to generate them — and, if the user wishes, combine data from multiple experiments.
Enjoy reading 91ÑÇÉ«´«Ã½ Today?
Become a member to receive the print edition four times a year and the digital edition weekly.
Learn moreGet the latest from 91ÑÇÉ«´«Ã½ Today
Enter your email address, and we’ll send you a weekly email with recent articles, interviews and more.
Latest in Science
Science highlights or most popular articles

From the journals: JBC
Huntington protein interactions affect aggregation. Intrinsically disordered protein forms a scaffold. From unknown protein to curbing cancer growth. Read about recent JBC papers on these topics.

An inclusive solar eclipse — with outreach
Traveling more than 150 miles with a group of neurodivergent students to have them witness a rare orbital alignment. and also teach the public about it, requires some strategic planning.

Predicting fatty liver disease from a tiny blood sample
Obesity and being overweight aren't the only factors that contribute to liver disease. New tests can help identify who is at risk or already has the disease, even in people who are lean or have a normal weight.

An ancient animal helps scientists improve modern technology
The same molecules that help tardigrades survive extreme weather can improve cryo-EM images of cellular structures and proteins, a team led by University of Wisconsin–Madison researcher Ci Ji Lim reports.

New structure gives insight into mRNA export and cancers
Yi Ren’s lab at Vanderbilt has described the structure of a protein complex that sheds light on the underlying molecular mechanism of mRNA export.

Analyzing triglycerides in Americans of African ancestry
Using the All of Us database, researchers at Vanderbilt sought a genetic reason why some patients, often underrepresented in research, could have varying levels of fat in the bloodstream.